Artificial intelligence is the flashy new thing in education today. Large language models, especially GPT-4, are already being used by millions of teachers. Student-facing applications built on those models are also becoming increasingly prevalent. But is it good for learning? As with any new breakthrough, the research will take some time to catch up. That’s why our curiosity at EdTech Evolved was piqued when a group of researchers from Microsoft published the results of one of the first large, pre-registered experiments exploring the efficacy of AI for math instruction.
Citation: Kumar, Harsh and Rothschild, David M. and Goldstein, Daniel G. and Hofman, Jake, Math Education with Large Language Models: Peril or Promise? (November 22, 2023). Available at SSRN: https://ssrn.com/abstract=4641653 or http://dx.doi.org/10.2139/ssrn.4641653
Experimental Design
Participants: 1,202 people from the Amazon Mechanical Turk workforce
Experiment: Subjects were randomly assigned to one of six possible conditions (see below). They were asked to complete two practice problems designed to mirror SAT math questions. Subjects then played a game for a one-minute break before completing two test problems. Lastly, subjects completed a survey with “feedback and additional self-reported measures.”
Possible Conditions:
- Subjects tried the practice problems first, before seeing the correct answer
- Subjects tried the practice problems first, before seeing the correct answer and a “stock” explanation from GPT-4
- Subjects tried the practice problems first, before seeing the correct answer and a customized explanation from GPT-4 based on a finely tuned pre-prompt with problem-solving strategies
- Subjects were shown the correct answer before attempting the practice problems
- Subjects were shown the correct answer before attempting the practice problems, along with a “stock” explanation from GPT-4
- Subjects were shown the correct answer before attempting the practice problems, along with a customized explanation from GPT-4 based on a finely tuned pre-prompt with problem-solving strategies
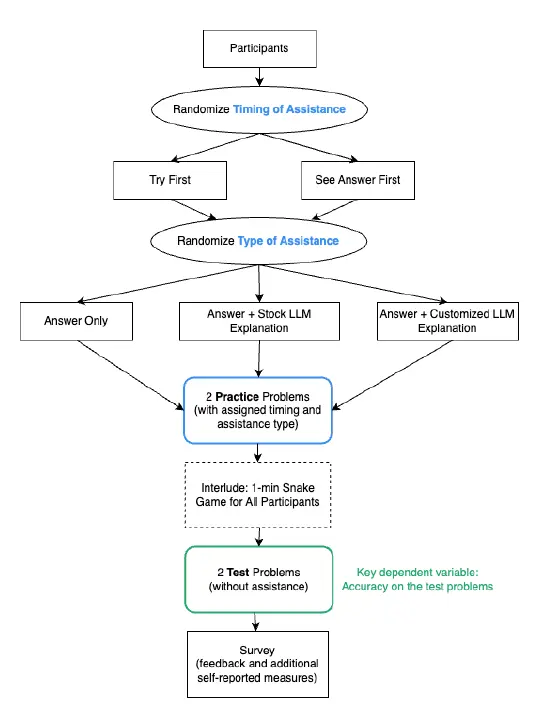
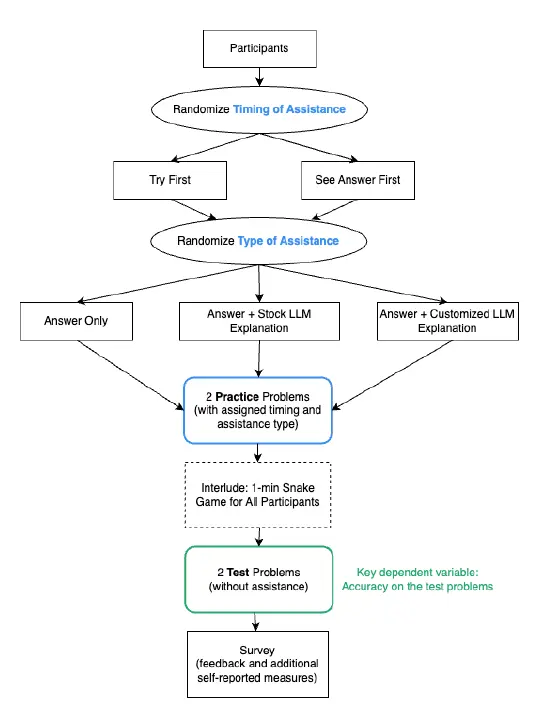
Study Results
Several insights on both instructional theory and the role of large language models emerged from this study, including:
- Subjects who tried the practice problems first before seeing the answer performed better on the test problems across all three conditions (answer only, stock GPT-4 explanations, and customized GPT-4 explanations).
- Subjects who tried the practice problems first and received GPT-4 explanations had accuracy rates more than 10% higher than those who were shown the answer first and received GPT-4 explanations.
- The approach subjects took to solving the test problems varied based on the type of explanation they were exposed to in the practice round.
- 72% of those who saw only the answer either guessed at the test problems or applied strategies other than what GPT-4 recommended.
- 51% of those who saw the stock explanations applied those strategies to their test problems.
- 47% who saw the customized explanations applied those strategies to their test problems.
- Subjects found the test problems to be significantly less difficult when they received a GPT-4 explanation as opposed to seeing the answer only.
- Subjects were far more likely to report feeling like they learned something when they received a GPT-4 explanation as opposed to seeing the answer only.
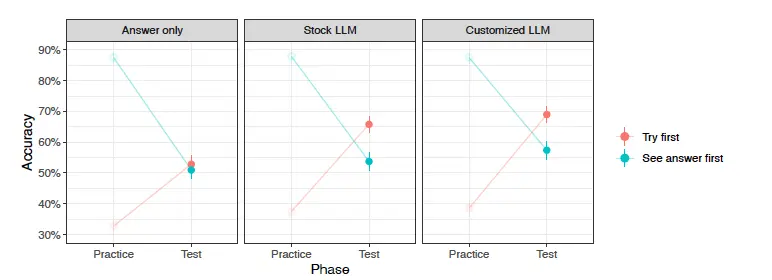
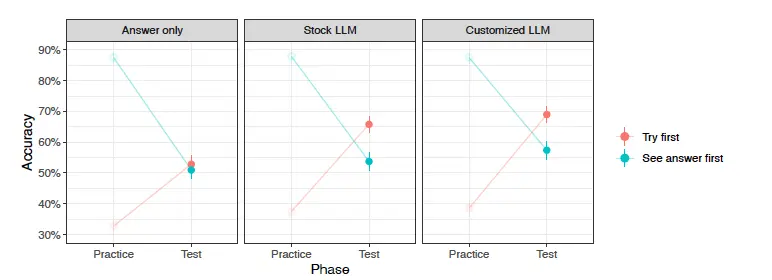
The two biggest takeaways on AI for math instruction:
- Validation of the concept of productive struggle. We don’t learn by seeing the answer to a problem. We learn by working through the process and applying the strategies, experience, and knowledge we pick up along the way.
- A strong indication that LLMs can add value in the role of real-time tutor. At least for the four types of math questions included in this experiment, GPT-4 was able to equip subjects with the strategies they needed to improve their results in a span of minutes. The customized version of GPT-4 helped students even more. The latter demonstrates the importance of “human-AI teaming” and keeping humans in the loop.
Potential Limitations
If history has taught us anything, it’s that we need to take great care not to overreact to every new published study. Here are some things to consider about this one:
- The researchers were all employed by or interning at Microsoft when the study was performed. Microsoft, like us at EdTech Evolved, has a vested interest in AI and is not an unbiased party. That’s not to infer that anything nefarious was going on. The experimental design looks strong and the results are aligned with much of what we already know about the value of feedback. This is just something to be aware of.
- The study was limited to four specific question types:
- Calculating the average speed of a multi-segment trip
- Solving for two unknowns with two constraints
- Determining the parity of an algebraic expression
- Identifying the missing measurement from an average
- GPT-4 “provided correct answers and coherent explanations” consistently for these question types. But, all LLMs are “known to make errors and provide incorrect explanations.” Were that to happen in a similar scenario, we could anticipate an adverse impact on learning.
- The experiment was limited solely to SAT-style math problems with multiple choice response formats. The researchers believed that some subjects were able to “game” the multiple-choice format without actually learning the content.
- The experiment was focused on “short-term learning and retention.” Subjects had just a one-minute break between practice and test problems.
- The pool of subjects was adequate in size, but not necessarily representative of real students in real-world scenarios.
Bonus: The Custom Prompt
Prompt engineering is a skill many of us will be fine-tuning for years to come. The research team for this study included their full customized prompt in the interest of transparency. This prompt is a great example of how teachers with strong instructional backgrounds and subject matter expertise can make LLMs like ChatGPT even more valuable by adding recommendations and context.
Note: The prompt was copied word-for-word from the study, including a couple of grammatical errors. We avoided correcting those in the interest of staying true to the research.
You are a tutor designed to help people understand and perform better on the types of problems you are given.
When solving problems where there are unknown numbers, try making up a values for those number to simplify the math. Choose these numbers so that any subsequent arithmetic works out nicely (e.g., by choosing numbers that are whole number multiples of all numbers mentioned in the problem to avoid decimal points or fractions in subsequent divisions).
Here are some strategies to use when arriving at and explaining answers,if applicable:
- When solving problems that involve speeds, emphasize the “D = RT” strategy for “Distance = Rate * Time”
- When solving problems that involve averages (other than speeds), emphasize the “T = AN” strategy for “Total = Average Number”
- When given a problem with two unknown numbers involving their sum and difference, suggest the strategy of starting with half their sum and distributing the difference
Explain how to solve the given problem in a way which makes it easiest for the learner to understand and remember so that they are able to apply it to a similar problem in future. Avoid solutions that require memorization of concepts and avoid complex notation. The learner should be able to develop some intuition to solve similar problems.
In the end, summarize the solution in minimal number of lines so that the learner is able to remember the method in future.
TL;DR – Summarizing the Study
Researchers at Microsoft conducted an online experiment with just over 1,200 subjects to determine whether LLM-generated explanations could help subjects perform better on SAT math problems. The research showed that LLM-generated explanations had a significant positive effect on the subjects’ accuracy. It also showed that subjects who attempted practice problems before being shown the answer scored higher on the test problems.
The group that experienced the most success was those who attempted the practice problem on their own, then were given an explanation of how to arrive at the right answer based on a customized GPT-4 prompt featuring known math strategies.
This study and others like it are foundational building blocks for the body of AI/LLM research still to come. Early results like this are encouraging and offer a strong indication that the most popular implementations of AI in schools to date are on the right track.
Stay Connected, Stay Informed
Keep yourself in the loop on all things AI in education, including the very latest research on the topic. Enter your email address below for monthly updates delivered to your inbox.